Agentic AI: The Future of Observability
- Scott Shultz
- Feb 24
- 16 min read
by Scott Shultz
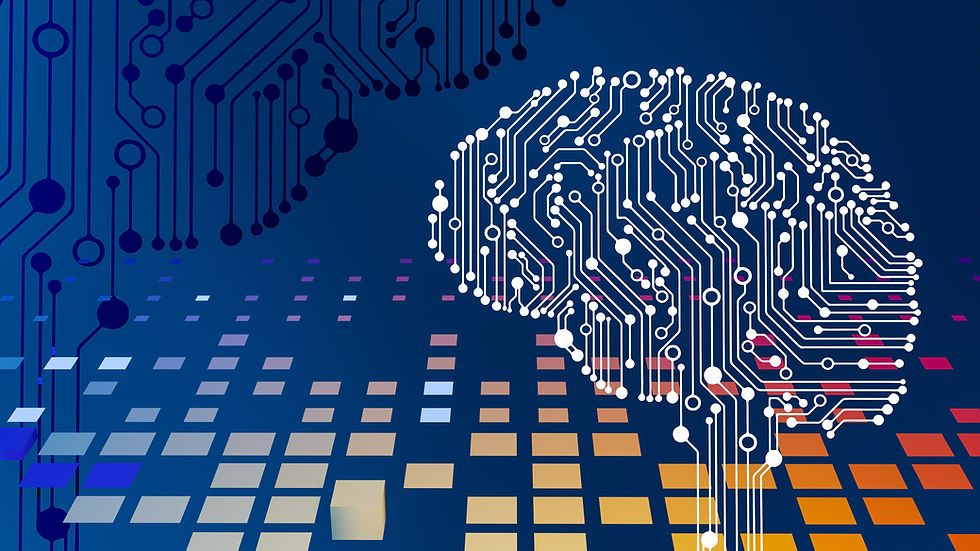
In the relentlessly evolving digital landscape, businesses are grappling with unprecedented complexity. Applications are distributed across hybrid and multi-cloud environments, microservices architectures are proliferating, and the volume, velocity, and variety of data generated are exploding. This intricate web of interconnected systems, while enabling innovation and agility, presents a formidable challenge: observability. Traditional monitoring approaches, designed for simpler, monolithic systems, are failing to provide the granular insights and proactive capabilities needed to navigate this complexity effectively.
Enter Agentic AI-Based Technology Observability. This paradigm shift moves beyond passive data collection and reactive alerting, embracing intelligent agents and artificial intelligence to actively observe, analyze, and act upon the vast streams of telemetry data emanating from modern IT environments. It's not just about seeing what's happening; it's about understanding why it's happening, predicting future issues, and autonomously taking corrective actions.
This comprehensive article delves into the core tenets of Agentic AI-Based Technology Observability, exploring its transformative potential across key dimensions:
Unlocking Actionable Business Insights:
Observability is not merely a technical discipline; its ultimate value lies in driving business outcomes. Agentic AI-based observability transcends traditional metrics-driven dashboards, providing contextually rich insights that directly impact strategic decision-making and operational efficiency.
From Technical Metrics to Business KPIs: Traditional monitoring often focuses on infrastructure-level metrics like CPU utilization, memory usage, and network latency. While crucial for technical teams, these metrics are often disconnected from business objectives. Agentic AI bridges this gap by correlating technical telemetry with business-relevant Key Performance Indicators (KPIs). For example, instead of just alerting on high latency in a database, an agentic system can correlate this latency with customer transaction failure rates, order processing delays, or revenue loss. This translates technical issues into tangible business impact, enabling leadership to prioritize incidents based on their actual consequences.
Contextualized Performance Analysis: Agentic systems leverage AI to understand the context surrounding performance data. They move beyond simple threshold-based alerts to identify anomalies, trends, and patterns that might be invisible to human operators. For instance, a slight increase in CPU utilization might be normal during peak hours, but anomalous and indicative of a potential issue during off-peak times. Agentic AI learns these baselines, understands seasonal variations, and identifies subtle deviations that point to underlying problems before they escalate into major outages.
Proactive Problem Identification and Prediction: By analyzing historical data and identifying emerging trends, agentic AI can predict potential future issues. Imagine an agentic system noticing a gradual increase in resource consumption in a specific microservice over time, correlating it with an upcoming marketing campaign that will drive increased traffic. The system can proactively alert operations teams about potential scaling needs before the campaign launches, preventing performance degradation and ensuring a seamless customer experience. This proactive approach drastically reduces reactive firefighting and allows businesses to anticipate and mitigate risks.
Enhanced Business Decision Making: The rich insights generated by agentic observability empower data-driven decision-making at all levels. Executive dashboards can display real-time business performance, highlighting areas of strength and weakness. Product teams can leverage performance data to understand user behavior, identify bottlenecks in user journeys, and optimize application features for improved user satisfaction. Operations teams can proactively allocate resources, optimize infrastructure spending, and streamline workflows based on intelligent recommendations from the agentic system.
Customer Experience Optimization: Ultimately, business success hinges on delivering exceptional customer experiences. Agentic observability plays a crucial role by providing end-to-end visibility into the customer journey. By monitoring application performance, user behavior, and infrastructure health across all touchpoints, agentic systems can identify friction points, latency issues, and error patterns that negatively impact customer satisfaction. For example, agentic agents can monitor real user metrics (RUM) in web applications and mobile apps, detecting slow page load times, JavaScript errors, or broken links that frustrate users and lead to cart abandonment or negative reviews. Addressing these issues proactively translates into improved customer loyalty, higher conversion rates, and increased revenue.
Cost Optimization and Resource Efficiency: Agentic observability can identify areas of resource wastage and inefficiencies. By analyzing resource utilization patterns across the infrastructure, agentic systems can pinpoint underutilized servers, idle processes, or inefficient code segments that are consuming unnecessary resources. This enables organizations to optimize infrastructure spending, right-size cloud resources, and improve application performance, leading to significant cost savings and increased operational efficiency.
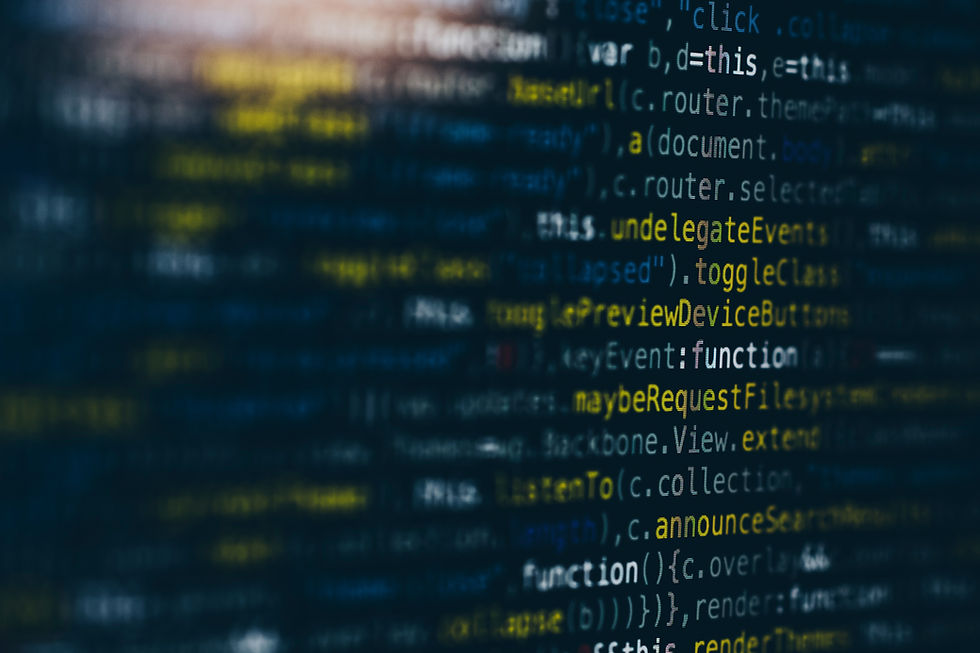
Multi-Source Ingestion: Embracing Data Diversity:
The complexity of modern IT environments necessitates a holistic observability approach that transcends siloed data sources. Agentic AI-based observability excels at ingesting and correlating data from a vast array of sources, painting a comprehensive picture of system behavior.
Diverse Data Source Integration: Agentic systems are designed to be inherently polyglot, capable of seamlessly integrating with a wide range of monitoring data sources. This includes:
Metrics: Time-series data representing numerical measurements like CPU utilization, memory usage, network bandwidth, request rates, error counts, and latency. Agentic agents can collect metrics from infrastructure components (servers, databases, containers), applications (JVM metrics, custom application metrics), and network devices.
Logs: Textual records of events, errors, warnings, and informational messages generated by applications and systems. Agentic log management capabilities enable efficient log aggregation, parsing, indexing, and search, allowing for rapid troubleshooting and root cause analysis.
Traces: Distributed tracing data, following requests as they propagate through complex, microservices-based architectures. Agentic tracing agents instrument applications to capture request flows, identify performance bottlenecks in specific services or components, and visualize end-to-end request latency.
Events: Discrete occurrences of significance, such as deployments, configuration changes, security alerts, and infrastructure scaling events. Agentic event management systems correlate events with performance data to understand the context surrounding incidents and identify contributing factors.
Synthetic Monitoring: Proactive simulations of user interactions and application workflows to identify potential issues before they impact real users. Agentic synthetic monitoring tools can simulate web application logins, API calls, transaction flows, and application availability checks from various geographical locations.
Real User Monitoring (RUM): Data collected from actual user interactions with web applications and mobile apps, providing insights into user experience, performance from different browsers and devices, and geographic distribution of users. Agentic RUM agents embedded in applications capture user behavior, page load times, JavaScript errors, and other relevant metrics.
Network Flow Data: Network traffic metadata that provides visibility into communication patterns, bandwidth utilization, and network performance across different network segments. Agentic network monitoring tools analyze flow data like NetFlow, sFlow, and IPFIX to identify network congestion, security threats, and connectivity issues.
Cloud Provider Metrics & Logs: Integration with cloud platforms like AWS, Azure, and GCP to ingest cloud-native metrics, logs, and events. Agentic systems can leverage cloud APIs to collect data from services like EC2, RDS, Lambda, Azure VMs, Azure SQL Database, and Google Compute Engine.
Custom Data Sources: Flexibility to ingest data from custom applications, legacy systems, and specialized monitoring tools through APIs, SDKs, and plugins. Agentic platforms often provide extensible architectures that allow users to define custom data ingestion pipelines and integrate with niche monitoring solutions.
Data Normalization and Harmonization: Data from diverse sources often arrives in varying formats and schemas. Agentic AI plays a crucial role in normalizing and harmonizing this data into a unified representation. This involves:
Schema Mapping: Automatically mapping data fields from different sources to a common schema, ensuring consistent data representation across the observability platform.
Data Transformation: Converting data into a uniform format, handling different data types, units of measurement, and time zones.
Semantic Enrichment: Adding contextual metadata to data points, such as service names, application versions, environment names, and geographical locations.
Data Deduplication and Cleansing: Removing redundant or inconsistent data, ensuring data quality and accuracy for analysis.
Intelligent Data Correlation and Contextualization: Simply ingesting data is not enough; the real power of agentic observability lies in its ability to correlate data from different sources and provide meaningful context. Agentic AI engines perform sophisticated data analysis to:
Identify Relationships: Discover dependencies and relationships between different entities in the IT environment, such as services, applications, databases, and infrastructure components.
Event Correlation: Group related events from different sources to identify incidents and understand their scope and impact.
Causal Analysis: Determine the root cause of performance issues by analyzing correlated data and identifying the sequence of events that led to the problem.
Topology Mapping: Dynamically construct and visualize the topology of the IT environment, showing dependencies and relationships between components based on observed data.
Scalable Data Ingestion and Processing: Modern IT environments generate massive volumes of telemetry data. Agentic observability platforms are designed to be highly scalable and performant, capable of:
High-Velocity Data Ingestion: Handling rapid data streams from thousands of sources, ensuring no data loss or bottlenecks.
Real-Time Data Processing: Analyzing data in near real-time to enable timely detection of issues and proactive responses.
Distributed Data Processing: Leveraging distributed computing architectures to process large datasets efficiently.
Efficient Data Storage and Retrieval: Employing optimized data storage solutions and indexing techniques to enable fast querying and analysis of historical data.
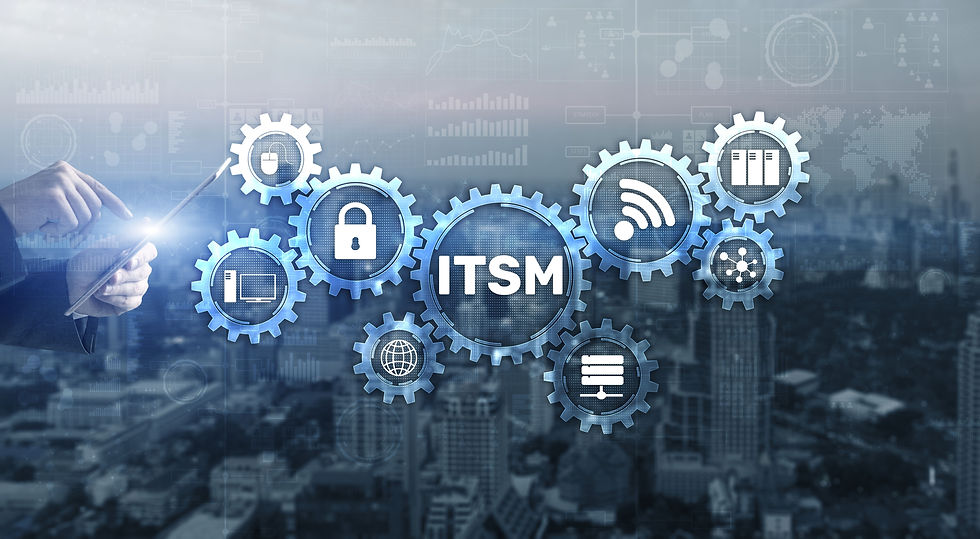
ITSM Integration - Bridging the Gap Between Observability and Action:
Observability data is most impactful when seamlessly integrated with existing IT Service Management (ITSM) workflows. Agentic AI-based observability acts as a powerful engine to automate ITSM processes, improve incident response, and enhance collaboration between operations and service management teams.
Automated Incident Detection and Alerting: Agentic systems go beyond basic threshold-based alerts to proactively identify incidents based on intelligent anomaly detection and pattern recognition. They can:
Context-Aware Alerting: Suppress noise and alert fatigue by generating alerts only for truly significant issues, considering context, severity, and business impact.
Intelligent Alert Routing: Automatically route alerts to the relevant teams or individuals based on service ownership, on-call schedules, and skill sets.
Automated Alert Enrichment: Attach relevant context to alerts, such as affected services, impacted users, potential root causes, and recommended actions, enabling faster troubleshooting.
Proactive Alert Generation: Predict potential incidents based on trend analysis and anomaly detection, alerting teams before issues escalate into major outages.
Automated Incident Creation and Ticketing: Upon detecting an incident, agentic systems can automatically create incident tickets in ITSM platforms like ServiceNow, Jira Service Management, or Remedy. This automation eliminates manual ticket creation, reduces response time, and ensures proper documentation of incidents. The automatically created tickets can be pre-populated with:
Incident Description: Detailed description of the incident, including symptoms, affected services, and potential impact.
Severity and Priority: Automatically assigned based on the business impact of the incident.
Affected Configuration Items (CIs): Identification of the CIs impacted by the incident, linking the incident to the CMDB.
Diagnostic Data and Logs: Relevant logs, metrics, traces, and diagnostic data attached to the ticket for faster troubleshooting.
Automated Remediation and Self-Healing: Agentic AI can go beyond detection and alerting to automate remediation actions. For recurring or well-defined issues, agentic systems can:
Trigger Automated Runbooks: Execute pre-defined scripts or workflows to automatically resolve common issues, such as restarting services, scaling resources, or rolling back deployments.
Self-Healing Capabilities: Implement self-healing mechanisms that automatically detect and resolve issues without human intervention, reducing downtime and improving system resilience.
Closed-Loop Remediation: Continuously monitor the system after remediation actions to verify effectiveness and ensure issues are fully resolved.
ITSM Workflow Automation: Agentic observability can automate various ITSM workflows beyond incident management, including:
Change Management: Automatically assess the risk and impact of proposed changes, provide recommendations for change approval, and monitor the performance of systems after changes are deployed.
Problem Management: Proactively identify recurring incidents and underlying problems, initiate problem investigations, and automate root cause analysis.
Knowledge Management: Automatically create knowledge base articles based on incident resolution steps, best practices, and troubleshooting guides.
Configuration Management: Continuously monitor configuration drift and automatically detect unauthorized changes, ensuring configuration compliance and security.
CMDB Integration and Contextual Awareness: Deep integration with Configuration Management Databases (CMDBs) enhances the context and accuracy of observability insights. Agentic systems can:
Dynamically Update CMDBs: Automatically update CMDBs with discovered infrastructure and application components, maintaining an up-to-date view of the IT environment.
Enrich Observability Data with CMDB Information: Leverage CMDB data to enrich observability data with configuration details, service dependencies, and ownership information.
Visualize Service Context: Provide context-aware dashboards and visualizations that show the relationship between performance data and CMDB information, allowing teams to understand the impact of incidents on specific services and applications.
Enhanced Collaboration and Communication: Agentic observability facilitates improved collaboration and communication between different teams involved in incident response and service management.
Shared Visibility: Provide a unified view of system health and performance across operations, development, and service management teams, fostering a shared understanding of issues.
Collaborative Troubleshooting: Enable teams to collaborate within the observability platform, sharing insights, annotations, and troubleshooting steps.
Automated Communication: Automate notifications and updates to stakeholders based on incident status, progress, and resolution, keeping everyone informed throughout the incident lifecycle.
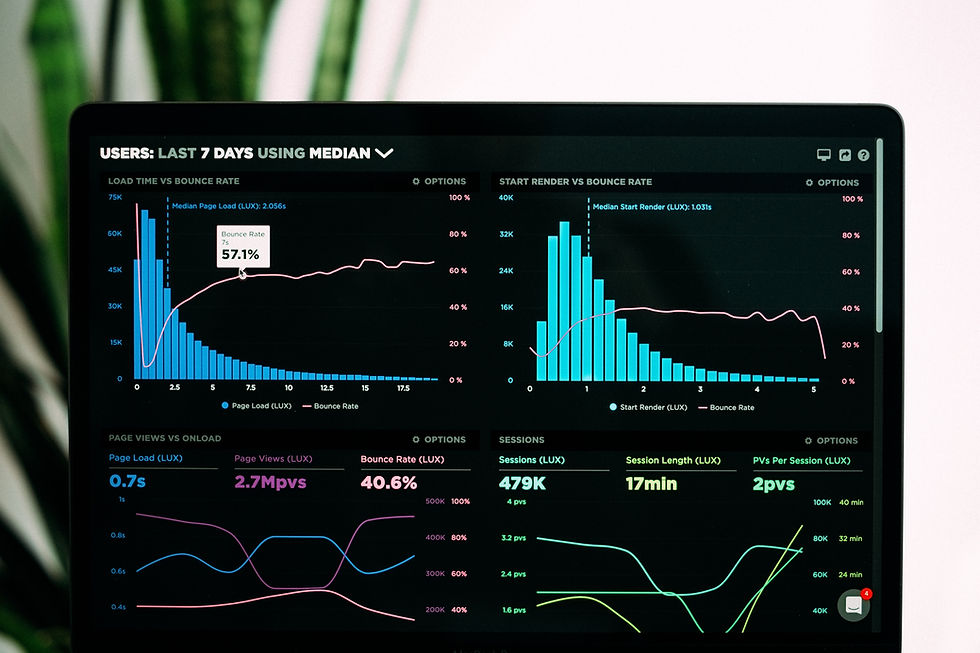
Unified Visibility: Breaking Down Silos for a Holistic Perspective
In complex, distributed environments, data silos are a major impediment to effective observability. Agentic AI-based observability champions unified visibility, bringing together data from disparate sources into a single, cohesive platform, enabling a holistic understanding of system behavior.
Single Pane of Glass for End-to-End Visibility: Agentic platforms provide a unified dashboard or interface that presents a consolidated view of all relevant observability data. This "single pane of glass" eliminates the need to switch between multiple monitoring tools and dashboards, providing a central hub for understanding system health. This unified view encompasses:
Infrastructure Visibility: Real-time monitoring of servers, networks, databases, containers, and cloud infrastructure.
Application Visibility: Performance monitoring of applications, microservices, APIs, and user transactions.
Business Transaction Visibility: Tracking business transactions across the entire IT ecosystem, from user requests to backend processes.
Security Visibility: Integration with security information and event management (SIEM) systems to correlate security events with performance data.
User Experience Visibility: Monitoring real user experience metrics to understand how users are interacting with applications and identify performance issues impacting users.
Context-Aware Dashboards and Visualizations: Agentic systems offer highly customizable and context-aware dashboards that go beyond static charts and graphs. These dashboards:
Dynamic Dashboards: Adapt to changing conditions and user needs, displaying relevant data based on context, user roles, and current incidents.
Interactive Visualizations: Employ interactive visualizations, such as heatmaps, topology maps, and geographical maps, to present complex data in an intuitive and digestible format.
Drill-Down Capabilities: Allow users to drill down into specific data points to investigate underlying details and understand the root cause of issues.
Customizable Views: Enable users to create personalized dashboards tailored to their specific roles and responsibilities, focusing on the metrics and data most relevant to them.
Dynamic Topology Mapping and Dependency Visualization: Agentic systems automatically discover and map the dynamic topology of the IT environment, visualizing dependencies between services, applications, and infrastructure components. This dynamic topology mapping:
Automated Discovery: Continuously discover and update the topology as the environment evolves, reflecting changes in infrastructure, application deployments, and service dependencies.
Dependency Visualization: Clearly visualize dependencies between components, showing how failures in one component can cascade and impact other parts of the system.
Service Context Mapping: Map applications and services to underlying infrastructure components, providing a holistic view of service health and dependencies.
Real-Time Topology Updates: Reflect changes in the topology in real-time, ensuring the visualization accurately represents the current state of the environment.
Correlation and Root Cause Analysis Across Silos: Unified visibility enables agentic AI to perform correlation and root cause analysis across previously siloed data sources. By analyzing data from metrics, logs, traces, events, and other sources in a unified context, agentic systems can:
Identify Causal Relationships: Pinpoint causal relationships between events and performance issues, determining the root cause of incidents that span multiple layers of the IT stack.
Reduce Mean Time to Resolution (MTTR): Accelerate troubleshooting by providing a comprehensive view of the incident context, enabling teams to quickly isolate the root cause and implement remediation actions.
Proactive Problem Identification: Identify emerging patterns and trends across different data sources, proactively detecting potential problems before they escalate into major incidents.
Eliminate Data Silos: Break down data silos between different monitoring tools and teams, fostering collaboration and shared understanding.
Role-Based Access Control and Data Security: While providing unified visibility, agentic platforms also incorporate robust role-based access control (RBAC) and data security mechanisms to ensure data privacy and compliance.
Granular Access Control: Define granular access controls based on user roles, teams, and data sensitivity, ensuring users only have access to the data relevant to their responsibilities.
Data Encryption: Encrypt data in transit and at rest to protect sensitive information from unauthorized access.
Audit Logging: Maintain comprehensive audit logs of user activities and data access, ensuring compliance with security and regulatory requirements.
Compliance Frameworks: Support compliance with industry regulations and security frameworks, such as GDPR, HIPAA, and SOC 2.
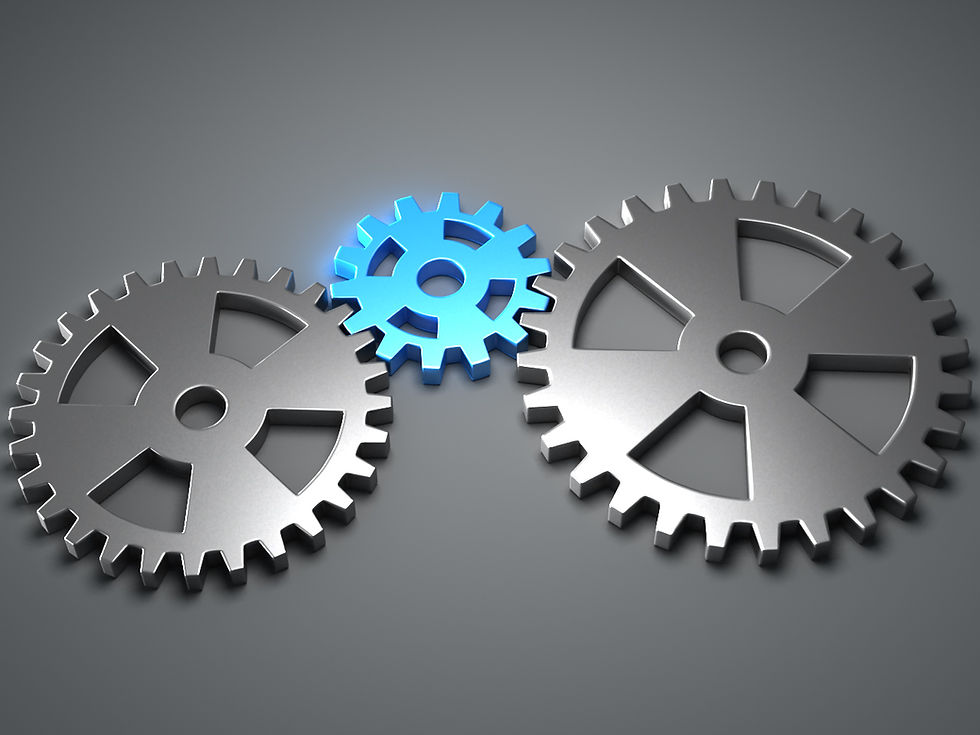
AI-Based Automation: Empowering Proactive and Autonomous Operations
Agentic AI-based observability's true power lies in its ability to drive automation. By leveraging AI and machine learning, these systems can automate a wide range of operational tasks, shifting from reactive monitoring to proactive and even autonomous operations.
Intelligent Anomaly Detection and Noise Reduction: Agentic AI algorithms learn normal system behavior and automatically detect anomalies that deviate from established baselines. This goes beyond simple threshold-based alerts by:
Dynamic Baselines: Establishing dynamic baselines that adapt to changing workload patterns, seasonality, and system evolution.
Contextual Anomaly Detection: Identifying anomalies in context, considering factors like time of day, day of week, and application deployments.
Noise Reduction: Filtering out noisy or irrelevant alerts, focusing attention on truly significant anomalies that require investigation.
Early Anomaly Detection: Detecting subtle anomalies early, before they escalate into major incidents, allowing for proactive intervention.
Automated Root Cause Analysis and Diagnostics: Agentic AI can automatically analyze correlated data to identify the root cause of performance issues and incidents. This automation:
Causal Inference: Employing AI algorithms to infer causal relationships between events and performance metrics, pinpointing the root cause of incidents.
Automated Diagnostics: Collect and analyze relevant diagnostic data, such as logs, traces, and configuration details, to understand the root cause and scope of the issue.
Reduced Troubleshooting Time: Significantly reduce the time spent on manual troubleshooting and root cause analysis, accelerating incident resolution.
Prioritized Remediation: Identify the most impactful remediation actions based on root cause analysis, ensuring teams focus on resolving the core problem.
Predictive Analytics and Capacity Planning: Agentic AI leverages historical data and trend analysis to predict future system behavior and resource needs. This predictive capability enables:
Resource Forecasting: Predicting future resource utilization, such as CPU, memory, storage, and network bandwidth, enabling proactive capacity planning.
Performance Prediction: Forecasting potential performance bottlenecks and degradation based on current trends and anticipated workload changes.
Proactive Scaling Recommendations: Providing recommendations for scaling resources up or down based on predicted demand, optimizing resource utilization and cost efficiency.
Risk Prediction: Identifying potential risks to system availability and performance based on emerging patterns and anomalies, allowing for proactive risk mitigation.
Automated Remediation and Self-Healing: As discussed in ITSM Integration, agentic AI can automate remediation actions for recurring or well-defined issues. This automation empowers systems to:
Autonomous Issue Resolution: Automatically resolve common issues without human intervention, improving system resilience and reducing downtime.
Self-Healing Infrastructure: Create self-healing infrastructure that can automatically detect and recover from failures, minimizing disruptions.
Runbook Automation: Execute automated runbooks or workflows to address known issues, streamlining incident response.
Continuous Optimization: Continuously optimize system performance and resource utilization through automated tuning and configuration adjustments.
AI-Powered Recommendations and Insights: Agentic AI systems go beyond automation to provide intelligent recommendations and actionable insights to human operators. This includes:
Recommended Actions: Suggesting optimal remediation actions based on root cause analysis and best practices, guiding operators towards effective solutions.
Performance Optimization Recommendations: Providing insights and recommendations for optimizing application performance, resource utilization, and configuration settings.
Security Threat Recommendations: Identifying potential security threats and vulnerabilities based on anomaly detection and security event correlation, recommending proactive security measures.
Contextual Guidance: Providing context-aware guidance and information to operators during incident response, accelerating troubleshooting and decision-making.
Continuous Learning and Improvement: Agentic AI systems are designed to continuously learn and improve their performance over time. Through machine learning and feedback loops, they:
Adaptive Anomaly Detection: Continuously refine anomaly detection models based on new data and feedback, improving accuracy and reducing false positives.
Improved Root Cause Analysis: Learn from past incidents and root cause analysis outcomes, improving the accuracy and speed of future root cause analysis.
Enhanced Automation Capabilities: Expand automation capabilities over time, automating more complex tasks and workflows as the system learns and matures.
Personalized Insights: Tailor insights and recommendations to individual user preferences and roles, improving relevance and usability.
Implementing Agentic AI-Based Technology Observability: A Practical Roadmap
Adopting agentic AI-based observability is not a flip-of-a-switch endeavor. It requires a strategic approach and a phased implementation. Here's a practical roadmap:
Define Clear Business Objectives: Start by clearly defining the business goals and desired outcomes for observability. What business KPIs do you want to improve? What operational efficiencies are you aiming for? What customer experience improvements are you targeting? Aligning observability initiatives with business objectives ensures that the effort is focused and delivers tangible value.
Assess Current Observability Maturity: Evaluate your current observability capabilities. What monitoring tools and systems are you using? What data sources are you currently collecting? How mature are your incident response processes? Identifying gaps and areas for improvement is crucial for a successful transition.
Identify Key Data Sources: Map out the key data sources relevant to your business objectives and IT environment. Consider metrics, logs, traces, events, synthetic monitoring, RUM, network flow data, and cloud provider data. Prioritize data sources that provide the most valuable insights and contribute to a holistic view.
Select the Right Agentic Observability Platform: Choose an agentic observability platform that aligns with your business requirements, technical environment, and budget. Evaluate platforms based on their capabilities in data ingestion, AI-powered analytics, ITSM integration, unified visibility, and automation. Consider factors like scalability, performance, security, and ease of use.
Start with a Pilot Project: Begin with a pilot project focused on a specific application, service, or business process. This allows you to test the chosen platform, validate its capabilities, and gain practical experience before a full-scale rollout. Focus the pilot on addressing a specific business challenge or demonstrating a clear ROI.
Implement Data Ingestion and Normalization: Configure data ingestion pipelines to collect data from identified sources. Implement data normalization and harmonization processes to ensure data quality and consistency. Leverage the platform's data integration capabilities to connect with diverse data sources.
Configure Anomaly Detection and Alerting: Configure intelligent anomaly detection algorithms and alerting rules based on your business requirements and established baselines. Fine-tune anomaly detection settings to minimize false positives and ensure alerts are actionable and context-aware.
Integrate with ITSM Systems: Integrate the agentic observability platform with your existing ITSM tools. Automate incident creation, ticket enrichment, and alert routing. Explore opportunities for ITSM workflow automation and closed-loop remediation.
Develop Unified Dashboards and Visualizations: Create context-aware dashboards and visualizations that provide a unified view of system health, application performance, and business KPIs. Customize dashboards for different user roles and teams, focusing on relevant data and insights.
Embrace AI-Powered Automation: Gradually introduce AI-powered automation capabilities, starting with automated diagnostics and root cause analysis. Explore opportunities for automated remediation and self-healing for recurring issues. Continuously refine automation workflows based on feedback and learning.
Foster a Culture of Observability: Promote a culture of observability across the organization. Educate teams on the benefits of agentic observability and empower them to leverage its insights for proactive problem solving, continuous improvement, and data-driven decision-making.
Iterate and Optimize: Continuously monitor the performance of the agentic observability platform, gather feedback from users, and iterate on configurations and automation workflows. Regularly evaluate the platform's ROI and adjust strategies as needed to maximize business value.
Challenges and Considerations
While Agentic AI-based Technology Observability offers immense potential, there are challenges and considerations to be aware of:
Data Volume and Complexity: Managing and processing massive volumes of data from diverse sources can be complex and resource-intensive. Platform scalability and efficient data processing are critical.
AI Model Training and Accuracy: AI models require training data and continuous refinement to ensure accuracy and effectiveness. Initial anomaly detection and root cause analysis may require adjustments and fine-tuning.
Integration Complexity: Integrating with legacy systems and diverse data sources can be challenging. Robust APIs, SDKs, and flexible data ingestion capabilities are essential.
Skills Gap: Implementing and managing agentic observability platforms requires specialized skills in AI, data science, observability principles, and ITSM. Addressing the skills gap through training and talent acquisition is crucial.
Security and Privacy: Protecting sensitive observability data and ensuring data privacy are paramount. Robust security controls, data encryption, and compliance with regulations are essential.
Cost Considerations: Agentic observability platforms can involve significant upfront and ongoing costs. Carefully evaluate the ROI and cost-benefit analysis before implementation.
The Future of Observability: Agentic AI as the Catalyst for Autonomous Operations
Agentic AI-based Technology Observability represents a fundamental shift in how we approach IT operations and business insights. It's moving us towards a future where systems are not just monitored, but actively observed, understood, and autonomously managed.
As AI and machine learning continue to advance, we can expect even more sophisticated agentic capabilities to emerge:
Generative AI for Observability: Generative AI models could be used to automatically generate dashboards, visualizations, and reports based on user queries and business objectives, further simplifying data consumption and analysis.
Hyper-Personalized Observability: Agentic systems could provide hyper-personalized observability experiences tailored to individual user roles and preferences, delivering highly relevant insights and recommendations.
Edge Observability: Extending agentic observability to the edge, monitoring and managing distributed edge devices and applications with AI-powered intelligence.
Autonomous Systems and Self-Optimizing Infrastructure: The ultimate vision is to create fully autonomous IT systems that can self-observe, self-diagnose, self-heal, and self-optimize, minimizing human intervention and maximizing resilience and efficiency.
Conclusion:
Agentic AI-based Technology Observability is not just a technological evolution; it's a strategic imperative for businesses navigating the complexities of the modern digital landscape. By embracing its core principles of business insights, multi-source monitoring, ITSM integration, unified visibility, and AI-powered automation, organizations can unlock unprecedented levels of operational efficiency, proactive problem solving, and data-driven decision-making. As we move towards increasingly complex and dynamic IT environments, agentic observability will be the cornerstone of resilient, agile, and customer-centric digital businesses. It's time to embrace the agentic revolution and unlock the full potential of your technology infrastructure to drive business success in the AI-powered era.
Comments